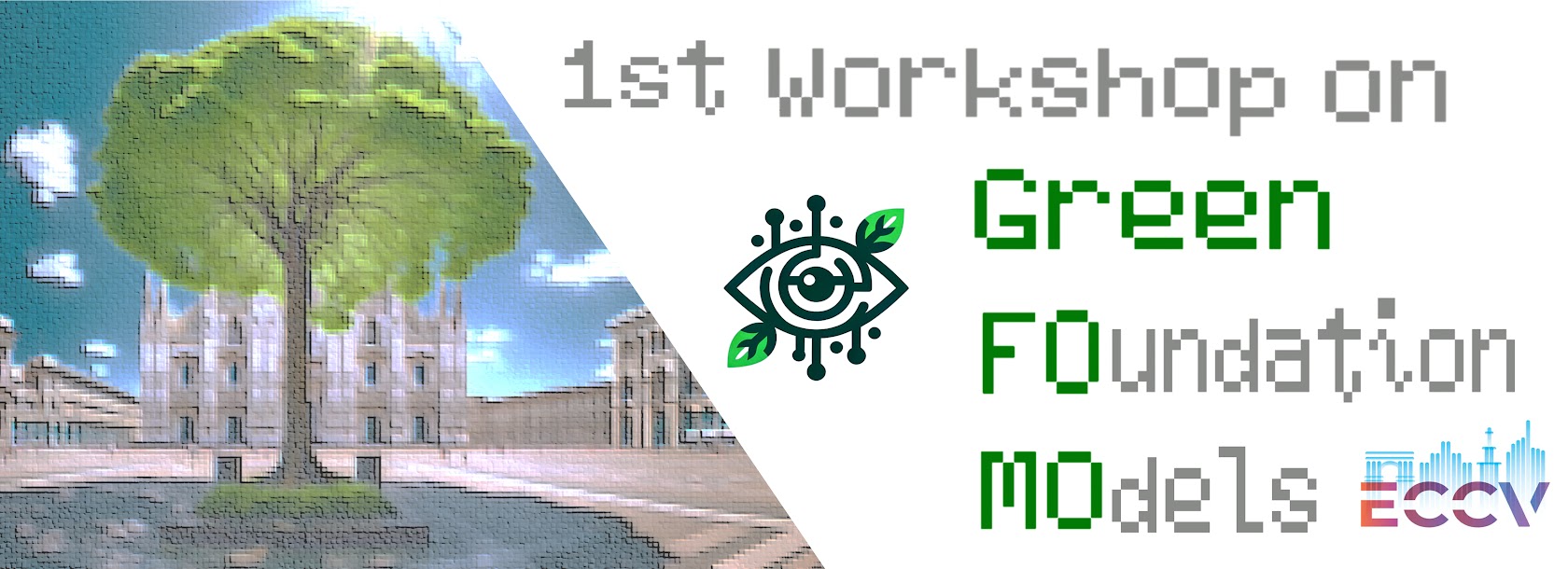
Introduction
Foundation models generalize well to various downstream tasks, thanks to their
web-scale pre-training, and have become a de-facto tool in pushing the frontiers of
computer vision research. Despite the exciting progress, developing foundation
models requires large compute resources, incurring heavy environmental costs.
For instance, CLIP reports the use of hundreds of GPUs and LLaMA 2 family led to an
emission of 539 tCO2eq, requiring about 27,000 trees in one year to capture the emissions.
To reduce computation and improve data efficiency, the computer vision community
has explored efficient ways of adapting large foundation models for downstream tasks.
For example, recent methods tune only a small set of learnable tokens
(or prompts) while keeping the weights of the pre-trained model frozen.
Compression methods also play a crucial role in optimizing the efficiency of
foundation models by pruning or distillation. Very recent methods have pioneered
in a new training-free paradigm by leveraging existing foundation models
or an assembly of them, to address computer vision tasks such as image
classification, 3D scene understanding, visual reasoning without fine-tuning.
Such research advancement is exciting and encouraging as it enables faster
adaptation to downstream tasks without excessive computation, while mitigating
the carbon footprint associated with resource-intensive processes.
The Green FOundation MOdels (GreenFOMO) workshop aims
to accelerate momentum around these emerging research topics, foster an inclusive
research and innovation ecosystem involving small/medium sized practitioners
in both academia and industry, and collectively making a green impact to society.
GreenFOMO promotes novel methodologies for efficient exploitation of
foundation models and encourage applications of FOMOs in domains that induce green
impacts, such as biodiversity, agricultural, food security, among others.
Invited Speakers
Dr. Ranjay Krishna is an Assistant Professor at the Paul G. Allen School of Computer Science & Engineering. His research lies at the intersection of computer vision and human computer interaction. This research has received best paper, outstanding paper, and orals at CVPR, ACL, CSCW, NeurIPS, UIST, and ECCV, and has been reported by Science, Forbes, the Wall Street Journal, and PBS NOVA. His research has been supported by Google, Amazon, Cisco, Toyota Research Institute, NSF, ONR, and Yahoo. He holds a bachelor's degree in Electrical & Computer Engineering and in Computer Science from Cornell University, a master's degree in Computer Science from Stanford University and a Ph.D. in Computer Science from Stanford University. His recent works cover instruction tuning for addressing complex visual tasks with low computational budget.
Dr. Elisabetta Farella is a researcher and head of the Energy Efficient Embedded Digital Architecture (E3DA) Research Unit at the Fondazione Bruno Kessler (FBK) in Trento, Italy. Her research focuses on smart sensing, embedded systems, and tinyML, emphasising energy-efficient and scalable machine-learning solutions for resource-constrained devices. The resource-aware, scalable AI solutions developed in her unit contribute to innovation across various national and international projects, engaging both industrial and academic sectors in advancing smart and energy-efficient technologies. Her publication record also reflects her contributions to IoT, wearable computing, and HCI domains, driving sustainable and innovative technological applications. Elisabetta recent work on energy-efficient embedded systems and TinyML is of great interest to the community.
Dr. Jose M. Alvarez is a research director at NVIDIA, leading the Autonomous Vehicle Applied Research team. His team maximizes the impact of the latest research advances on the AV product. Jose research interests include model-centric and data-centric deep learning toward more efficient and scalable systems. Jose completed his Ph.D. in computer science in Barcelona, specializing in road-scene understanding for autonomous driving when datasets were very limited. He also worked as a postdoctoral researcher at NYU under Yann LeCunn. Jose recent research on pruning and efficient deep learning will contribute to the workshop discussion.
Dr. Eric Schulz is an incoming professor at LMU and the director of the Institute of Human Centered AI at Helmholtz Munich. He finished PhD at UCL in 2017 working on generalization and exploration in reinforcement learning. From 2017 to 2019, he was a Data Science Postdoctoral Fellow at Harvard University, where he worked on computational models of learning and decision making and from 2020 to 2023 he was a Max Planck Independent Group Leader at the MPI for Biological Cybernetics. His recent studies on LLMs will bring valuable insights to our workshop from a cognitive perspective.
Dr. Judy Hoffman is assistant Professor in the School of Interactive Computing at Georgia Tech and a member of the Machine Learning Center. Research interests include computer vision, machine learning, domain adaptation, robustness, and fairness. Prior to joining Georgia Tech, Dr. Hoffman was a Visiting Research Scientist at Facebook AI Research and a postdoctoral scholar at Stanford University and UC Berkeley. She received her PhD from UC Berkeley, EECS in 2016 where she was a member of BAIR and BDD. Her recent works focus on FOMO efficiency, e.g. Token Merging (ToMe) and Binary Vision Transformers (BiViT) to reduce the computational burden.
Organizers
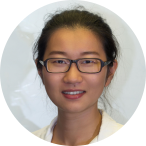
Fondazione Bruno Kessler
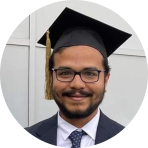
University of Trento
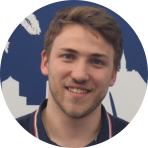
University of Trento
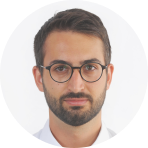
Fondazione Bruno Kessler
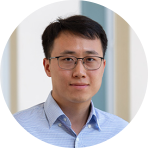
Hong Kong Baptist University
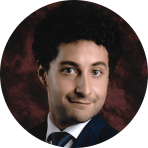
Télécom Paris, Institut Polytechnique de Paris
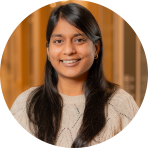
University of Montreal, Mila
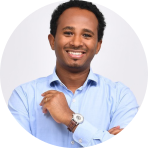
Microsoft AI for Good Research Lab
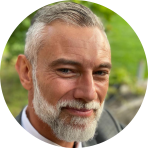
University of Verona
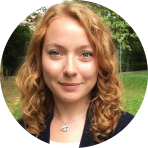
Technical University of Munich, Helmholtz Munich
Contact
To contact the organizers please use greenfomo*at*googlegroups*dot*com
Acknowledgments
Thanks to Robust Computer Vision Across Geographies Workshop for the webpage format.